The discovery and investigation of proteins is essential in life science, as proteins carry out a wide range of functions in living organisms. It has been proved that the 3-dimensional structure is a useful indicator of function, even more useful than the sequence in some cases.
In this page, you can discover your own enzyme from our integrated database consist of both public and our in-house genomic datasets.
The work flow is as follows,
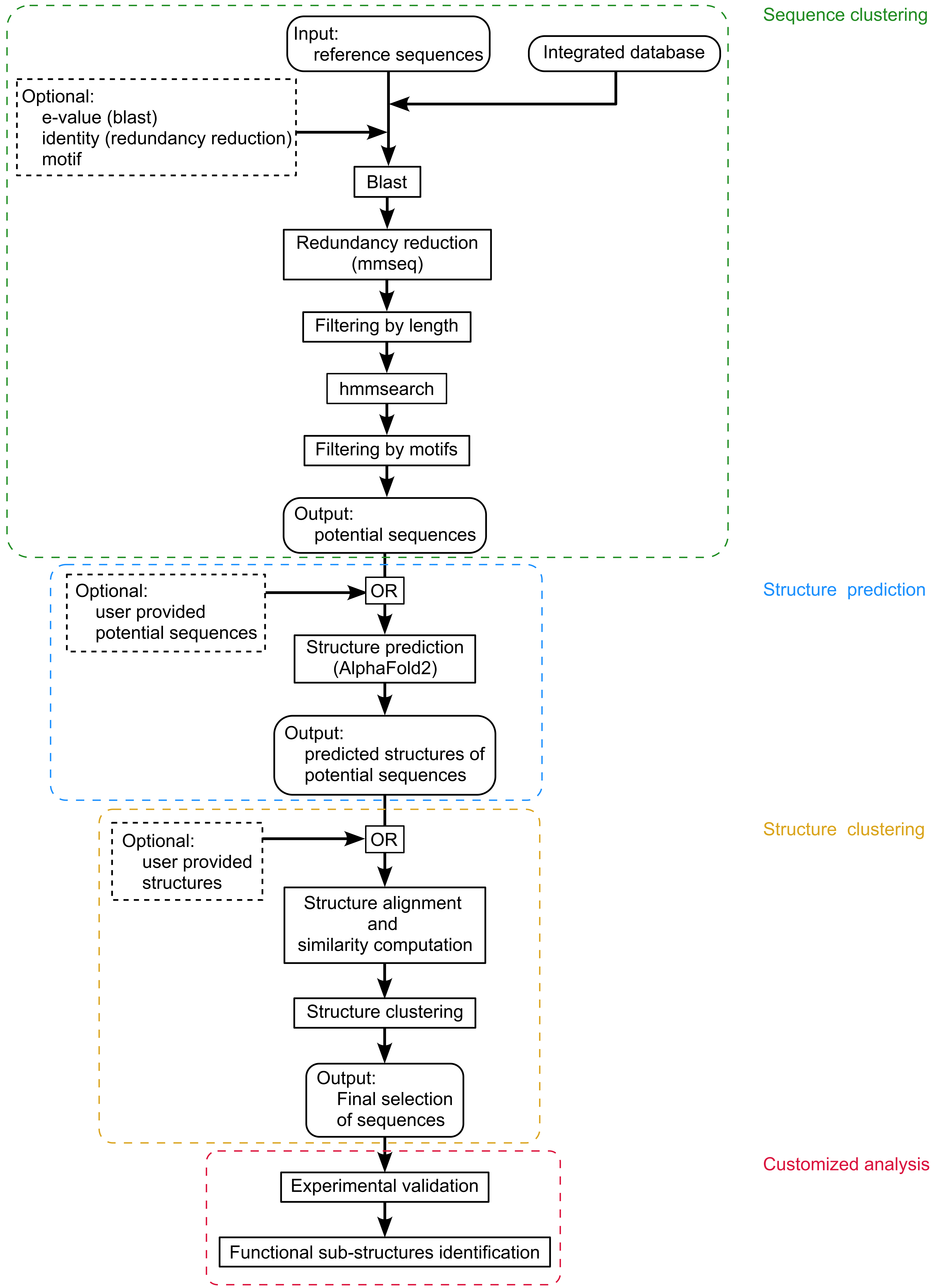
In the sequence clustering step, potential sequences are filtered from an integrated database according to the user provided reference sequences, where the integrated database consists of both public available and our in-house genomic databases. Optionally, one can specify the e-value used for blast search, the identity of sequences used for redundancy reduction, and provide several motifs as well.
In the structure prediction step, users can either provide or use the output from the sequence clustering step as the input. Three dimensional structures are predicted using AlphaFold2 (Jumper, John, et al., 2021).
In the structure clustering step, users can either provide or use the output from the structure prediction step as the input. Structure-based alignment and clustering are done here and then the final selection of sequences are provided.
Finally, contact us if customized analysis is needed, which includes experimental validation by BGI and further identification of functional sub-structure using our in-house developed algorithms.
In the following, we show a demo case about our discovery process of polyethylene terephthalate hydrolase (PETase), including a common 3-step analysis and a customized analysis with prior knowledge of experimental validation.
In the sequence clustering step, we selected in total 3200 sequences from our integrated database by specifying the motif and length. Here we show the tree graphics clustering according to the sequence similarity.
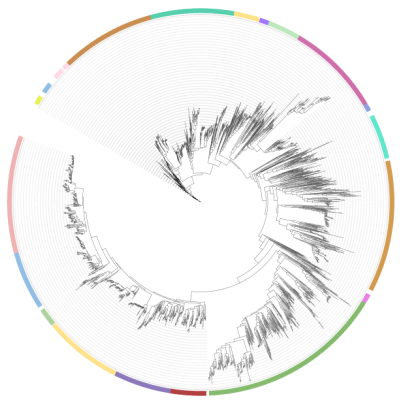
In the structure prediction step, we predicted structures of all 3200 sequences from the sequence clustering step using AlphaFold2.
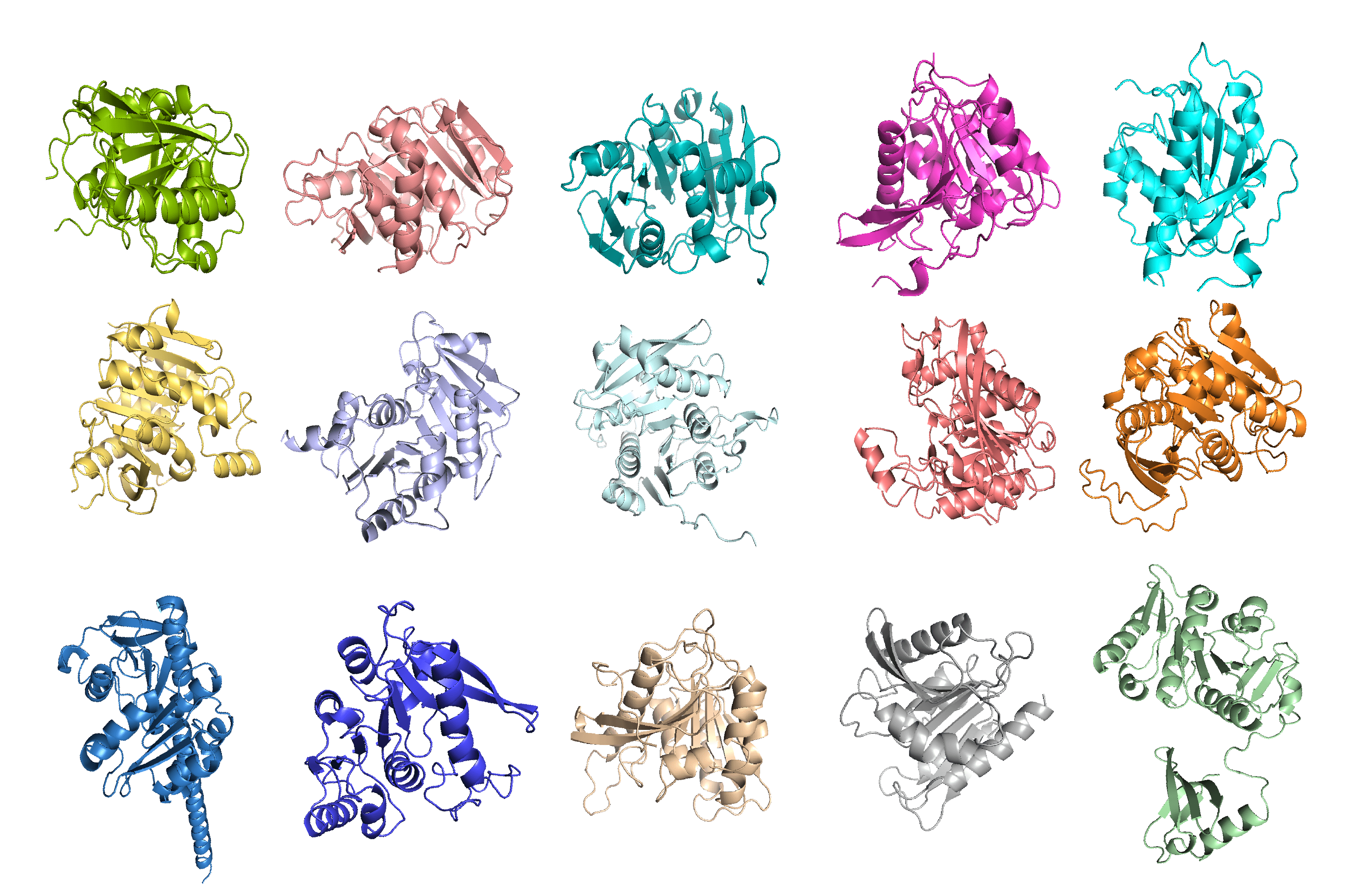
In the structure clustering step, we did structure-based clustering. Here we show the clustering results of both sequence-based and structure-based methods respectively. We then choose 2930 sequences from the 21 clusters for further experimental validation.
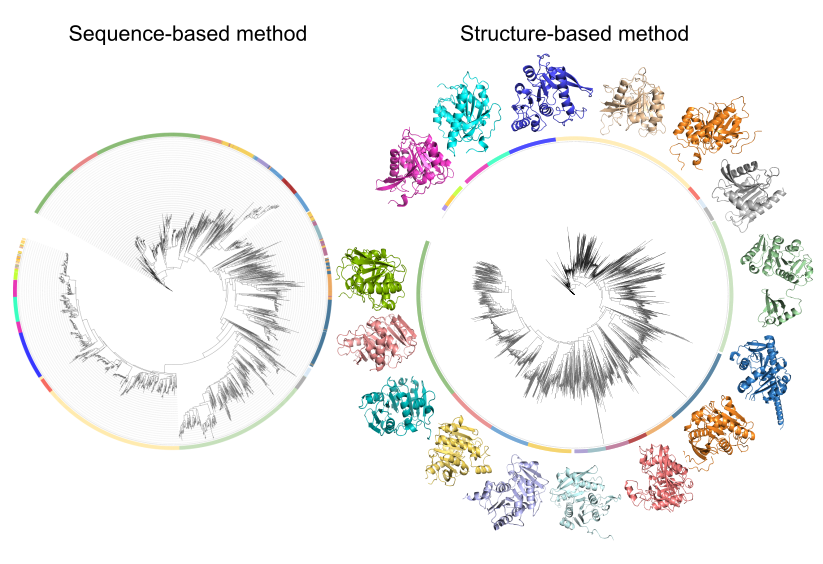
Here we show the experimental results of the PETase activity with different concentrations of NaCl. It is shown that even parts of the activate PETases are clustered together, the overall distribution is still kind of scattered.
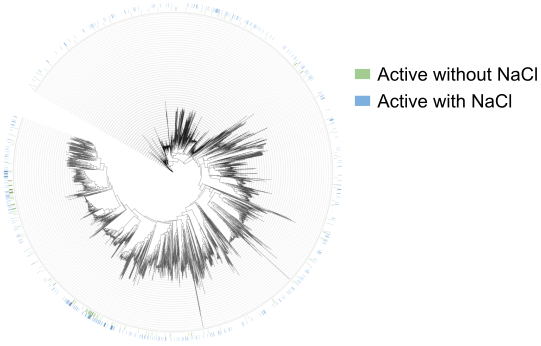
Here we show the clustering result of our newly developed method based on sub-structures. We generated two clusters, where most active PETases are clustered together.
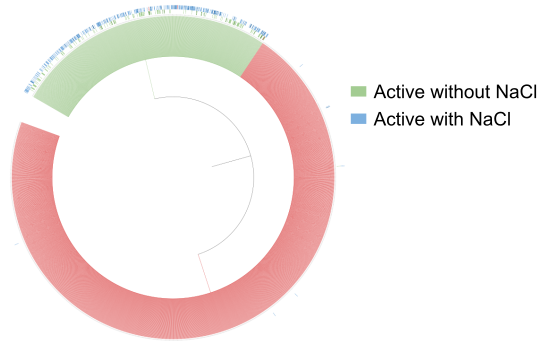
Here we show the discovered functional sub-structures (left). Where red color shows the catalytic triad, while the residue pairs with the same colors indicate potential interaction related to enzyme activity. The potential residue types related to enzyme activity (right) shows that serine plays an important role in the function of PETase.
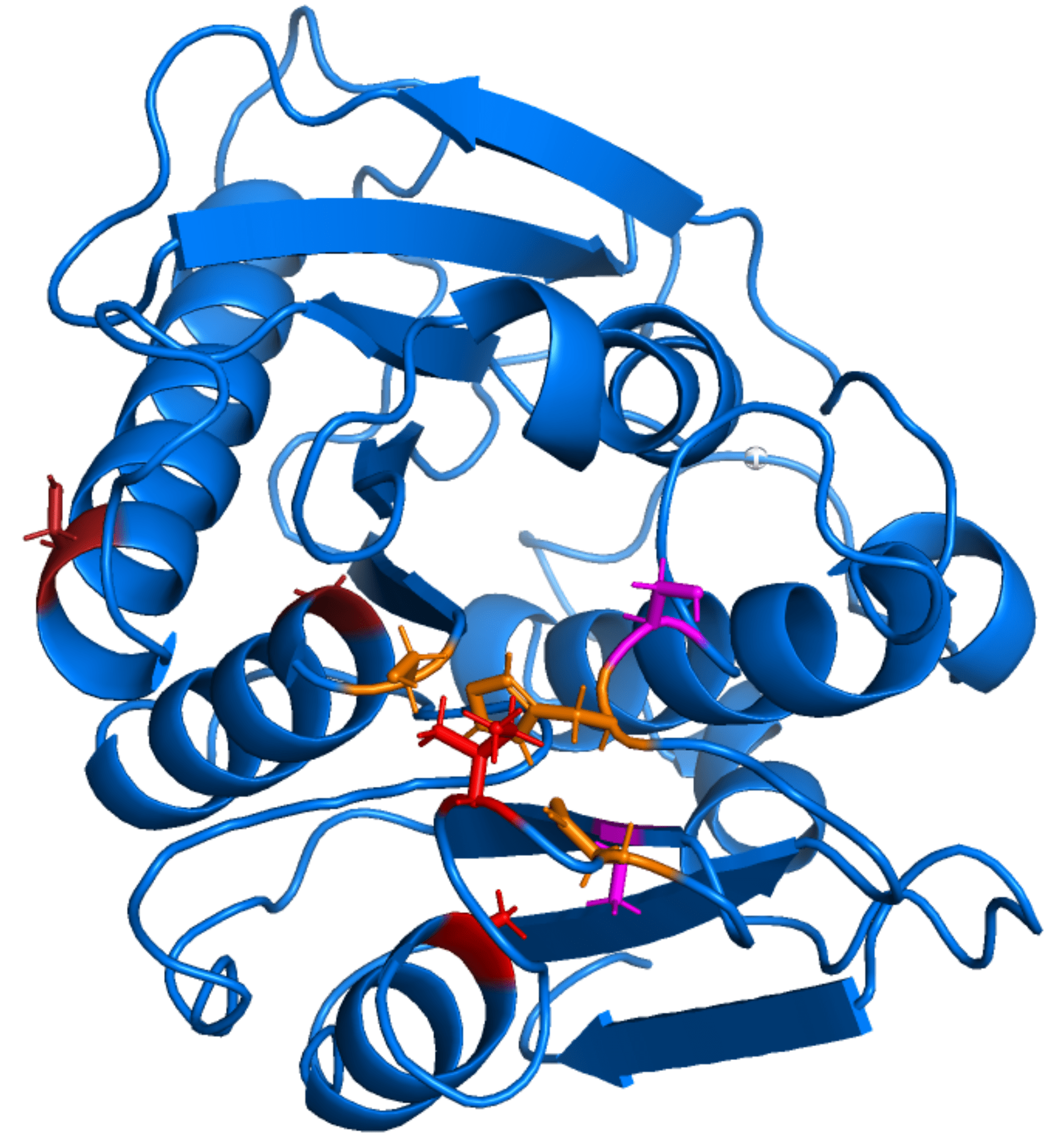